
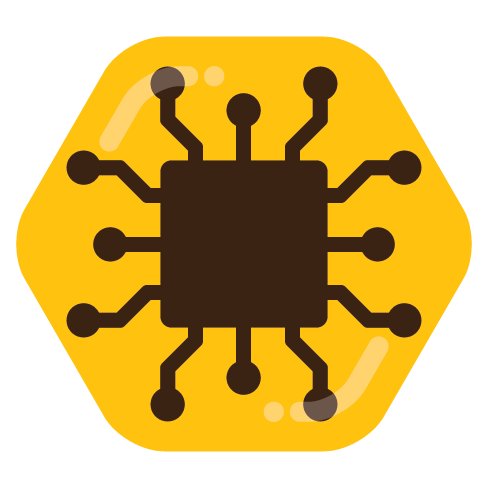
That’s not what lossless data compression schemes do:
In lossless compression the general idea is to create a codebook of commonly occuring patterns and use those as shorthand.
For example, one of the simplest and now ancient algorithms LZW does the following:
- Initialize the dictionary to contain all strings of length one.
- Initialize the dictionary to contain all strings of length one.
- Emit the dictionary index for W to output and remove W from the input.
- Add W followed by the next symbol in the input to the dictionary.
- repeat
Basically, instead of rewriting long sequences, it just writes down the index into an existing dictionary of already seen sequences.
However, once this is done, you now need to find an encoding that takes your characterset (the original characters+the new dictionary references) and turns it into bits.
It turns out that we can do this optimally: Using an algorithm called Arithmetic coding we can align the length of a bitstring to the amount of information it contains.
“Information” here meaning the statistical concept of information, which depends on the inverse likelihood a certain character is observed.
Logically this makes sense:
Let’s say you have a system that measures earthquakes. As one would expect, most of the time, let’s say 99% of the time, you will see “no earthquake”, while in 1% of the cases you will observe “earthquake”.
Since “no earthquake” is a lot more common, the information gain is relatively small (if I told you “the system said no earthquake”, you could have guessed that with 99% confidence: not very surprising).
However if I tell you “there is an earthquake” this is much more important and therefore is worth more information.
From information theory (a branch of mathematics), we know that if we want to maximize the efficiency of our codec, we have to match the length of every character to its information content. Arithmetic coding now gives us a general way of doing this.
However, we can do even better:
Instead of just considering individual characters, we can also add in character pairs!
Of course, it doesn’t make sense to add in every possible character pair, but for some of them it makes a ton of sense:
For example, if we want to compress english text, we could give a separate codebook entry to the entire sequence “the” and save a ton of bits!
To do this for pairs of characters in the english alphabet, we have to consider 26*26=676
combinations.
We can still do that: just scan the text 600 times.
With 3 character combinations it becomes a lot harder 26*26*26=17576
combinations.
But with 4 characters its impossible: you already have half a million combinations!
In reality, this is even worse, since you have way more than 26 characters: you have things like ", . ? !
and your codebook ids which blow up the size even more!
So, how are we supposed to figure out which character pairs to combine and how many bits we should give them?
We can try to predict it!
This technique, called [PPM](Prediction by partial matching) is already very old (~1980s), but still used in many compression algorithms.
The important trick is now that with deep learning, we can train even more efficient estimators, without loosing the lossless property:
Remember, we only predict what things we want to combine, and how many bits we want to assign to them!
The worst-case scenario is that your compression gets worse because the model predicts nonsensical character-combinations to store, but that never changes the actual information you store, just how close you can get to the optimal compression.
The state-of-the-art in text compression already uses this for a long time (see Hutter Prize) it’s just now getting to a stage where systems become fast and accurate enough to also make the compression useful for other domains/general purpose compression.
Have a look at Kraken which has many state-of-the-art models for both HTR and OCR