
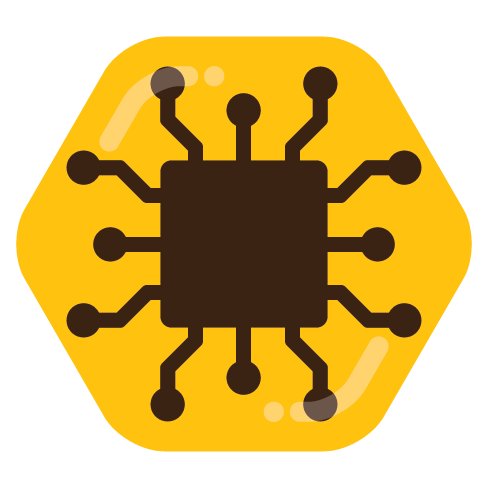
Deep learning has always been classified as AI. Some consider pathfinding algorithms to be AI. AI is a broad category.
AGI is the acronym you’re looking for.
Deep learning has always been classified as AI. Some consider pathfinding algorithms to be AI. AI is a broad category.
AGI is the acronym you’re looking for.
This feels to me like the LLM misinterpreted it as some kind of fictional villain talk and started to autocomplete it.
Could also be the model simply breaking. There was a time when Sydney (Bing AI or whatever they call it now) had to be constrained to 10 messages per context and having some sort of supervisor on top of itself because it would occasionally throw a fit or start threatening the user for no reason.
The author’s suggesting that smart people are more likely to fall for cons that they try to dissect but can’t find the specific method being used, supposedly because they consider themselves to be infallible.
I disagree with this take. I don’t see how that thought process is exclusive to people who are or consider themselves to be smart. I think the author is tying himself into a knot to state that smart people are actually the dumb ones, likely in preparation to drop an opinion that most experts in the field will disagree with.
The paracausal tarrasque seems like a genuinely interesting concept. Gives me False Hydra vibes
Have you tried Cosmoteer? It’s a pretty satisfying shipbuilder with resource and crew management, trading, and quests. Similar vibe to Reassembly.
So you’re basically saying that, in your opinion, tensor operations are too simple of a building block for understanding to ever appear out of them as an emergent behavior? Do you feel that way about every mathematical and logical operation that a high school student can perform? That they can’t ever in whatever combination create a system complex enough for understanding to emerge?
I don’t think that anyone would argue that the general public can even solve a mathematical matrix, much less that they can only comprehend a stool based on going down a row in a matrix to get the mathematical similarity between a stool, a chair, a bench, a floor, and a cat.
LLMs rely on billions of precise calculations and yet they perform poorly when tasked with calculating numbers. Just because we don’t calculate anything consciously to get a meaning of a word doesn’t mean that no calculations are actually done as part of our thinking process.
What’s your definition of “the actual meaning of the concept represented by a word”? How would you differentiate a system that truly understands the meaning of a word vs a system that merely mimics this understanding?
technology fundamentally operates by probabilisticly stringing together the next most likely word to appear in the sentence based on the frequency said words appeared in the training data
What you’re describing is Markov chain, not an LLM.
So long as a model has no regard for the actual you know, meaning of the word
It does, that’s like the entire point of word embeddings.
Your opening sentence is demonstrably false. GTP-2 was a shitpost generator, while GPT-4 output is hard to distinguish from a genuine human. Dall-E 3 is better than its predecessors at pretty much everything. Yes, generative AI right now is getting better mostly by feeding it more training data and making it bigger. But it keeps getting better and there’s no cutoff in sight.
That you can straight-up comment “AI doesn’t get better” at a tech literate sub and not be called out is honestly staggering.
I don’t think your assumption holds. Corporations are not, as a rule, incompetent - in fact, they tend to be really competent at squeezing profit out of anything. They are misaligned, which is much more dangerous.
I think the more likely scenario is also more grim:
AI actually does continue to advance and gets better and better displacing more and more jobs. It doesn’t happen instantly so barely anything gets done. Some half-assed regulations are attempted but predictably end up either not doing anything, postponing the inevitable by a small amount of time, or causing more damage than doing nothing would. Corporations grow in power, build their own autonomous armies, and exert pressure on governments to leave them unregulated. Eventually all resources are managed by and for few rich assholes, while the rest of the world tries to survive without angering them.
If we’re unlucky, some of those corporations end up being managed by a maximizer AGI with no human supervision and then the Earth pretty much becomes an abstract game with a scoreboard, where money (or whatever is the equivalent) is the score.
Limitations of human body act as an important balancing factor in keeping democracies from collapsing. No human can rule a nation alone - they need armies and workers. Intellectual work is especially important (unless you have some other source of income to outsource it), but it requires good living conditions to develop and sustain. Once intellectual work is automated, infrastructure like schools, roads, hospitals, housing cease to be important for the rulers - they can give those to the army as a reward and make the rest of the population do manual work. Then if manual work and policing through force become automated, there is no need even for those slivers of decency.
Once a single human can rule a nation, there is enough rich psychopaths for one of them to attempt it.
There are also other AI-related pitfalls that humanity may fall into in the meantime - automated terrorism (e.g. swarms of autonomous small drones with explosive charges using face recognition to target entire ideologies by tracking social media), misaligned AGI going rogue (e.g. the famous paperclip maximizer, although probably not exactly this scenario), collapse of the internet due to propaganda bots using next-gen generative AI… I’m sure there’s more.
I’d honestly go one step further and say that the problem cannot be fully solved period.
There are limited uses for voice cloning: commercial (voice acting), malicious (impersonation), accessibility (TTS readers), and entertainment (porn, non-commercial voice acting, etc.).
Out of all of these only commercial uses can really be regulated away as corporations tend to be risk averse. Accessibility use is mostly not an issue since it usually doesn’t matter whose voice is being used as long as it’s clear and understandable. Then there’s entertainment. This one is both the most visible and arguably the least likely to disappear. Long story short, convincing enough voice cloning is easy - there are cutting-edge projects for it on github, written by a single person and trained on a single PC, capable of being run locally on average hardware. People are going to keep using it just like they were using photoshop to swap faces and manual audio editing software to mimic voices in the past. We’re probably better off just accepting that this usage is here to stay.
And lastly, malicious usage - in courts, in scam calls, in defamation campaigns, etc. There’s strong incentive for malicious actors to develop and improve these technologies. We should absolutely try to find a way to limit its usage, but this will be eternal cat and mouse game. Our best bet is to minimize how much we trust voice recordings as a society and, for legal stuff, developing some kind of cryptographic signature that would confirm whether or not the recording was taken using a certified device - these are bound to be tampered with, especially in high profile cases, but should hopefully somewhat limit the damage.
Github link for convenience
https://github.com/Anuken/Mindustry
GPT3 is pretty bad at it compared to alternatives (although it’s hard to compete with calculators on that field), but if it was just repeating after the training dataset it would be way worse. From the study I’ve linked in my other comment (https://arxiv.org/pdf/2005.14165.pdf):
On addition and subtraction, GPT-3 displays strong proficiency when the number of digits is small, achieving 100% accuracy on 2 digit addition, 98.9% at 2 digit subtraction, 80.2% at 3 digit addition, and 94.2% at 3-digit subtraction. Performance decreases as the number of digits increases, but GPT-3 still achieves 25-26% accuracy on four digit operations and 9-10% accuracy on five digit operations, suggesting at least some capacity to generalize to larger numbers of digits.
To spot-check whether the model is simply memorizing specific arithmetic problems, we took the 3-digit arithmetic problems in our test set and searched for them in our training data in both the forms “<NUM1> + <NUM2> =” and “<NUM1> plus <NUM2>”. Out of 2,000 addition problems we found only 17 matches (0.8%) and out of 2,000 subtraction problems we found only 2 matches (0.1%), suggesting that only a trivial fraction of the correct answers could have been memorized. In addition, inspection of incorrect answers reveals that the model often makes mistakes such as not carrying a “1”, suggesting it is actually attempting to perform the relevant computation rather than memorizing a table.
In my comment I’ve been referencing https://arxiv.org/pdf/2005.14165.pdf, specifically section 3.9.1 where they summarize results of the arithmetic tasks.
That’s not entirely true.
LLMs are trained to predict next word given context, yes. But in order to do that, they develop internal model that minimizes error across wide range of contexts - and emergent feature of this process is that the model DOES perform more than pure compression of the training data.
For example, GPT-3 is able to calculate addition and subtraction problems that didn’t appear in the training dataset. This would suggest that the model learned how to perform addition and subtraction, likely because it was easier or more efficient than storing all of the examples from the training data separately.
This is a simple to measure example, but it’s enough to suggests that LLMs are able to extrapolate from the training data and perform more than just stitch relevant parts of the dataset together.
Was this ever a thing? I have never seen or heard anyone use “gen AI” to mean AGI. In fact I can’t even find one instance of such usage.